Invited Speaker
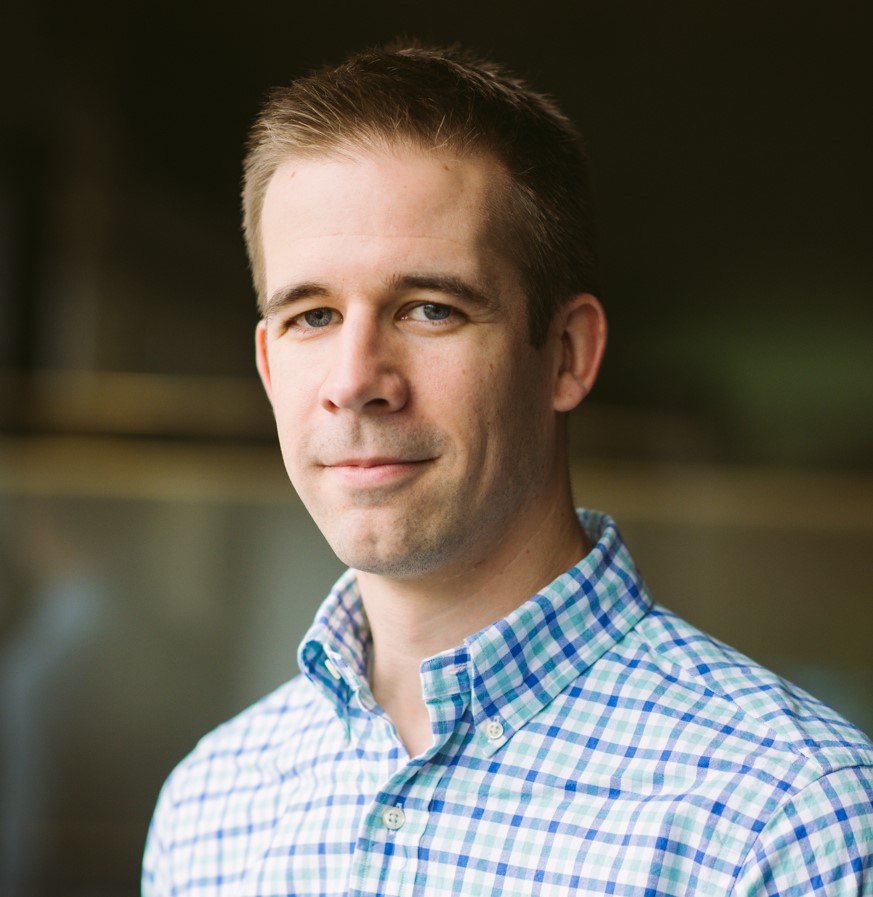
Asst. Prof. John Quilty
Department of Civil and Environmental EngineeringUniversity of Waterloo
Waterloo, Ontario, Canada
Speech Title: A stochastic data-driven framework for hydrological and water resources modelling
Abstract: Any hydrological model is subject to numerous uncertainty sources (input data, parameters, model output, etc.) that when ignored, leaves out valuable information that could otherwise be used to assess the model’s reliability. While it is becoming more common in hydrology and water resources for uncertainty to be considered in the modelling chain, there is a lack of frameworks that can holistically (and simply) tie these various sources together.
This talk will introduce a new data-driven framework that merges machine learning with a blueprint for converting deterministic models into stochastic ones, enabling numerous uncertainty sources to be considered in hydrological and water resources models. The result is a probabilistic framework that can be used for generating simulations or forecasts of any hydrological and water resources variable. Using two different case studies, special variants of this framework will be explored by considering time series pre-processing, model ensembles, and coupling with process-based models, demonstrating the improved performance of the framework against benchmark methods.
Keywords: Hydrological modelling, data-driven models, stochastics, uncertainty